Jessica Sampson
1 Year Ago
In today's economic landscape, being able to collect outstanding debt is more critical than ever for businesses and financial institutions alike. In an era of readily available technology, it is baffling that most companies are still relying on traditional debt collection techniques that often fall short in terms of both efficiency and effectiveness. In this blog, I will give an overview of how machine learning can be used to streamline the debt collection process and maximize recovery rates.
Rethinking Traditional Approaches
Traditionally, debt collection strategies have revolved around segmenting the population into two or three key dimensions, such as debt amount or length of delinquency. The problem with this approach is that it is after the event has already occurred, which significantly decreases the chances of recovery and does not provide insights as to the reason the delinquency occurred in the first place. Further, it ignores customers’ propensity to remedy organically, resulting in wasting resources on customers that would have repaid had they been given more time.
Unleashing Machine Learning Insights
Machine learning algorithms can process historical debt collection data to identify current patterns, trends, and correlations that would not be possible to detect manually. By using machine learning, businesses can gain valuable insights into customer behavior, payment patterns, and risk profiles and identify at-risk accounts BEFORE they become delinquent. These insights can also be clustered into look-alike segments to develop personalized strategies for each debtor.
Predicting Successful Debt Recovery
The first task of a machine learning methodology for debt collection is to predict the likelihood of successful debt recovery for every delinquent account. This is achieved by analyzing historical data and incorporating various factors such as payment history, demographic information, debit and credit transactions, and economic indicators, to generate accurate predictions about the probability of debt repayment. This empowers debt collection agencies to prioritize their efforts on debtors with a higher likelihood of repayment, resulting in cost minimization to proper resource allocation and higher recovery rates.
Tailoring Approaches Through Segmentation
Segmenting debtors based on their characteristics and behavior is crucial for tailoring debt collection approaches. Machine learning algorithms can categorize debtors into different segments based on numerous factors such as age, income, payment history, and debt amount. By identifying common characteristics among debtors, businesses can create personalized collection strategies and payment plans that resonate with each segment.
Furthermore, machine learning can seamlessly be used to perform sentiment analysis by analyzing unstructured data such as customer service calls recordings, posts on social platforms, emails, and review to understand the customer’s emotional state. By being able to detect signs of frustration, dissatisfaction, or willingness to cooperate, the communication strategy can also be adjusted in its tone, language, and timing. All these factors working together will significantly increase the chances of a positive outcome.
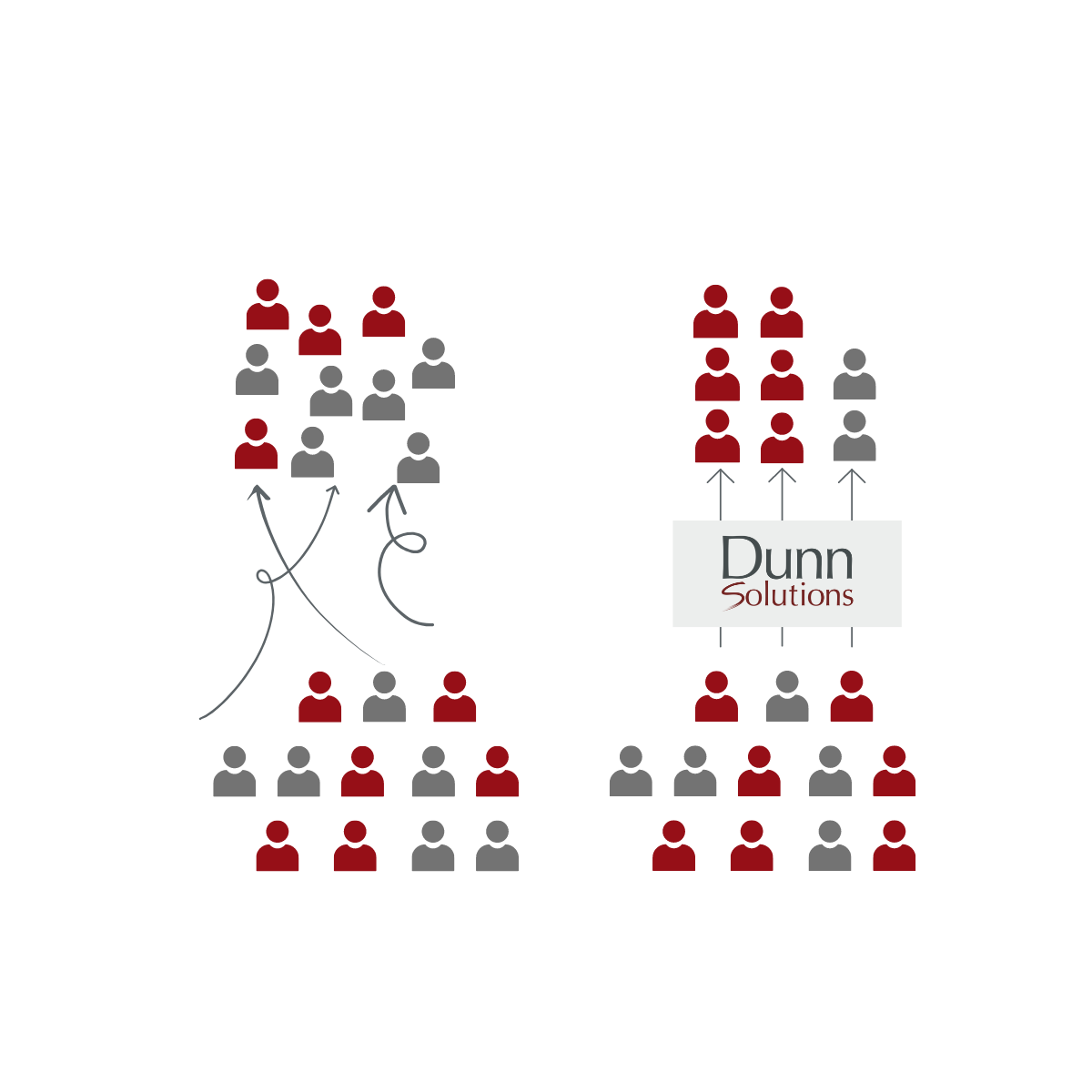
Proactive Risk Detection and Intervention
The last task of an effective machine learning strategy is to identify customers who are at risk of becoming delinquent before they do. Using the insights from the previous two steps, machine learning can detect warning signs and alert debt collectors before delinquency occurs. Proactive intervention using personalized communication with alternative financial solutions, such as negotiation of repayment plans, is often more cost-effective and helps support a positive relationship with the customer.
Unlocking the Potential of Machine Learning
Machine learning presents an incredible and yet vastly untapped opportunity for the debt collection industry. By harnessing the power of data mining, propensity modeling, customer segmentation, sentiment analysis, and early risk detection, businesses can optimize their debt collection strategies and maximize their recovery rates.
Dunn solutions has successfully implemented these solutions for major players in the financial industry, achieving as much as 3X increase in collection amounts and slashing costs by as much as 30%.
Blogtag
machine learning, debt collection, predictive analytics